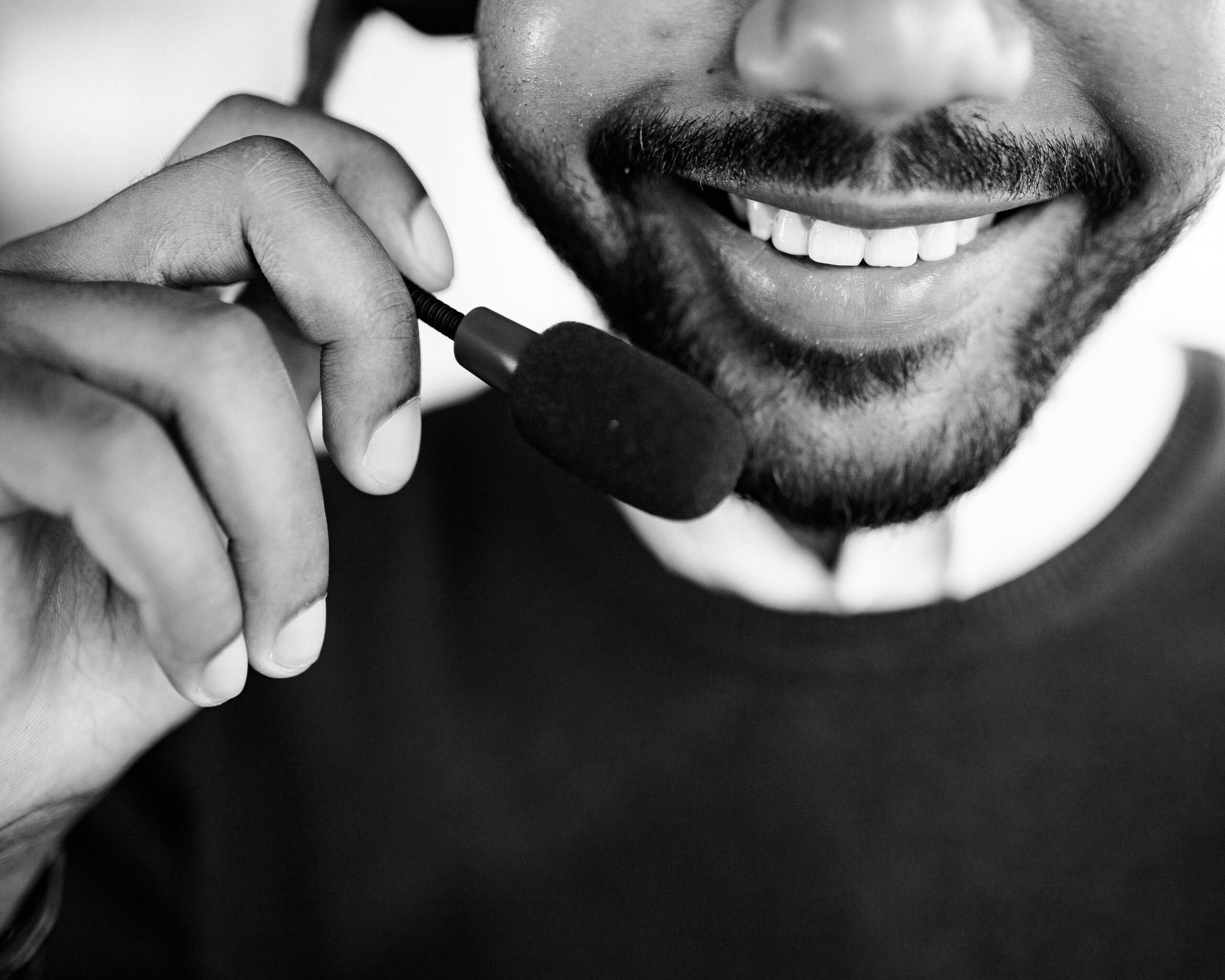
Understanding Customer Sentiment: AI Call Analysis in Action
- calltrack.ai
- No Comments
In the age of digital transformation, businesses are increasingly leveraging artificial intelligence (AI) to gain insights and improve customer experiences. One of the most transformative applications of AI in this regard is in analyzing customer sentiment through call analysis. Understanding customer sentiment can provide invaluable insights into customer satisfaction, preferences, and pain points, ultimately driving better decision-making and fostering stronger customer relationships.
The Importance of Customer Sentiment
Customer sentiment refers to the emotions and attitudes expressed by customers during interactions with a business. It encompasses a range of feelings, from satisfaction and happiness to frustration and disappointment. Understanding customer sentiment is crucial for several reasons:
- Customer Satisfaction: By analyzing sentiment, businesses can gauge how satisfied their customers are with their products or services. This helps in identifying areas that need improvement and recognizing what is working well.
- Customer Retention: Sentiment analysis can highlight potential issues before they escalate, allowing businesses to address problems proactively and retain customers.
- Product Development: Insights gained from sentiment analysis can inform product development by identifying features that customers love or dislike, helping businesses to better meet their customers’ needs.
- Brand Reputation: Monitoring sentiment can help in managing a brand’s reputation by detecting negative trends early and allowing for timely intervention.
The Role of AI in Sentiment Analysis
AI-driven sentiment analysis involves the use of natural language processing (NLP) and machine learning (ML) algorithms to analyze spoken or written language and determine the sentiment behind it. This technology can process vast amounts of data quickly and accurately, providing real-time insights that would be impossible to obtain manually.
Natural Language Processing
NLP is a branch of AI that focuses on the interaction between computers and human language. It enables machines to understand, interpret, and respond to human language in a valuable way. In the context of call analysis, NLP can transcribe spoken words into text, identify keywords and phrases, and analyze the overall sentiment of the conversation.
Machine Learning
Machine learning algorithms can be trained on large datasets to recognize patterns and make predictions. In sentiment analysis, ML models can be trained to classify segments of text or speech as positive, negative, or neutral based on the context and the specific words used. Over time, these models improve in accuracy as they are exposed to more data.
AI Call Analysis in Action
Let’s explore how AI-driven call analysis works in practice and the benefits it offers to businesses.
Transcription and Sentiment Detection
The first step in AI call analysis is transcribing the audio from customer calls into text. Advanced speech recognition technology is used to ensure high accuracy in transcription, even in noisy environments or with speakers who have strong accents.
Once the transcription is complete, the text is analyzed for sentiment. The AI system examines various linguistic features, such as tone, pitch, word choice, and context, to determine the sentiment expressed by the customer. This analysis is often broken down into smaller segments to capture shifts in sentiment throughout the conversation.
Real-Time Analysis and Alerts
One of the significant advantages of AI call analysis is the ability to provide real-time insights. As calls are being analyzed, the system can generate alerts for specific sentiment changes. For example, if a customer expresses frustration or anger, the system can notify a supervisor to intervene promptly. This real-time capability allows businesses to address issues immediately, improving customer satisfaction and preventing negative experiences from escalating.
Trend Analysis and Reporting
Beyond individual calls, AI call analysis can aggregate data from multiple interactions to identify broader trends. Businesses can generate reports that highlight common themes, frequently mentioned issues, and overall sentiment trends over time. This information is invaluable for strategic decision-making, helping businesses to prioritize resources and focus on areas that will have the most significant impact on customer experience.
Case Study: Customer Support
Consider a customer support center that handles thousands of calls daily. Implementing AI call analysis can revolutionize their operations in several ways:
- Improved Training: By analyzing calls, the support center can identify common customer pain points and train agents to handle these issues more effectively. Additionally, sentiment analysis can be used to assess agent performance, providing targeted feedback and coaching.
- Enhanced Customer Experience: Real-time sentiment analysis enables supervisors to monitor calls and intervene when necessary. This ensures that customers receive timely and appropriate responses, enhancing their overall experience.
- Operational Efficiency: Trend analysis helps the support center identify recurring issues that may indicate underlying problems with products or services. Addressing these issues proactively can reduce call volume and improve efficiency.
Challenges and Considerations
While AI-driven sentiment analysis offers numerous benefits, there are also challenges and considerations to keep in mind:
- Accuracy: Ensuring the accuracy of sentiment analysis can be challenging, particularly with complex or ambiguous language. Continuous training and refinement of ML models are necessary to maintain high accuracy.
- Privacy and Security: Analyzing customer calls involves handling sensitive data. Businesses must ensure that their AI systems comply with privacy regulations and have robust security measures in place to protect customer information.
- Integration: Integrating AI call analysis with existing systems and workflows can be complex. Businesses need to ensure seamless integration to maximize the benefits of the technology.
The Future of AI Call Analysis
The field of AI-driven sentiment analysis is continuously evolving. Advances in NLP and ML are making these systems more sophisticated and accurate. In the future, we can expect even more powerful tools that provide deeper insights and greater automation.
Personalization and Customization
Future AI systems will likely offer more personalized and customizable analysis. Businesses will be able to tailor sentiment analysis models to their specific industry, products, and customer base, resulting in more relevant and actionable insights.
Multilingual Support
As global businesses interact with customers from diverse linguistic backgrounds, multilingual support will become increasingly important. AI systems will continue to improve in their ability to analyze sentiment across different languages and dialects.
Integration with Other Channels
AI call analysis will extend beyond phone calls to include other communication channels, such as emails, chat, and social media. This holistic approach will provide a comprehensive view of customer sentiment across all touchpoints.
Embracing the Future of Customer Insights with AI Call Analysis
AI-driven call analysis is a powerful tool for understanding customer sentiment and improving customer experiences. By leveraging NLP and ML, businesses can gain real-time insights, identify trends, and make data-driven decisions that enhance customer satisfaction and loyalty. While challenges remain, the future of AI call analysis is bright, promising even more sophisticated and effective solutions for businesses worldwide. As AI technology continues to advance, the potential for transforming customer interactions and driving business success will only grow.